One of the key themes in the 2005 seminal paper by John Ioannidis “Why most published research findings are false” is that positive predictive value of a study depends not only on power but even more so on prior odds of a study hypothesis being true. Therefore, if the prior odds are low, “surprising” results of the study are said to have low positive predictive value.
While we know how to estimate statistical power (or, better to say, we know methods to do power calculations), likelihood of a study hypothesis being true is difficult to estimate and even more difficult to quantify.
For example, over the past several decades a very large number of chemically distinct dopamine D2 receptor antagonists were found to inhibit amphetamine-induced hyperactivity in laboratory rodents. Thus, if someone aims to test a new dopamine D2 receptor antagonist (bioavailable, CNS-penetrant, with adequate half-life, etc.), prior probability of inhibiting amphetamine-induced hyperactivity in common strains of laboratory rodents is rather high (or even very high). Therefore, when reported, such results will be judged as having high positive predictive value.
In contrast, an example of 5G cellular technologies facilitating spreading of SARS-CoV2 is at the opposite extreme (yes, there is a publication on that!).
But what about all the cases between such extremes?
We often discuss the example of an SOD1 mouse model where, if studies are designed with due rigor and according to standards described by Scott et al (2008), nearly all tested drugs so far fail to affect survival. Thus, when advancing a new drug into this kind of testing, we have to assume a prior probability being rather low and treat positive results with caution.
But what about a situation when there are LOTS of poorly designed studies with nearly exclusively positive results? An interesting example of such a case is provided by human microbiota-associated murine models that, according to a recent systematic review, are found to work essentially all the time (LINK). Do the principles of dialectic materialism rule here and the large quantity of low-rigor research translates into high-quality evidence?
Likely not. Walter et al. found that 95% of studies they reviewed reported transfer of the pathological phenotype, which they suggest is implausible and go on to posit that lack of experimental rigor is a major factor in this excess of ‘positive’ results. This is supported by a recent study (Yap et al., 2021) looking at gut microbiome and autism in which a large study failed to reproduce data from numerous previous underpowered studies.
In fact, corollary #6 in the Ioannidis 2005 paper states: “The hotter a scientific field (with more scientific teams involved), the less likely the research findings are to be true.” And the following text explains that, albeit paradoxical at the first glance, “… the PPV of isolated findings actually decreases when many teams of investigators are involved in the same field. This may explain why we occasionally see major excitement followed rapidly by severe disappointments in fields that draw wide attention. With many teams working on the same field and with massive experimental data being produced, timing is of the essence in beating competition.”
As Walter et al asked: ‘Where are the negative studies?’. We will probably never know if they outnumber the published positive findings, but literacy in good experimental design and a healthy dose of skepticism is clearly needed when evaluating exciting findings and before they are tested properly, in well designed and sufficiently powered studies.
We suggest a rough guide to how to estimate positive predictive value of reported findings when planning your own research (e.g., when estimating the sample sizes; see the companion case study below).
Figure 1 illustrates the joint operation of scientific odds and technical odds (risks of bias) in defining the likelihood a scientific hypothesis being true (i.e., what John Ioannidis defined as “the ratio of the number of “true relationships” to “no relationships” among those tested in the field”). Thus, for new exciting, but surprising and unprecedented research outcomes, which might even go against the current dogma in their field of research, one should seek protection against any risk of bias by adhering to the highest quality standards to be able to achieve reasonable chances of results being true.
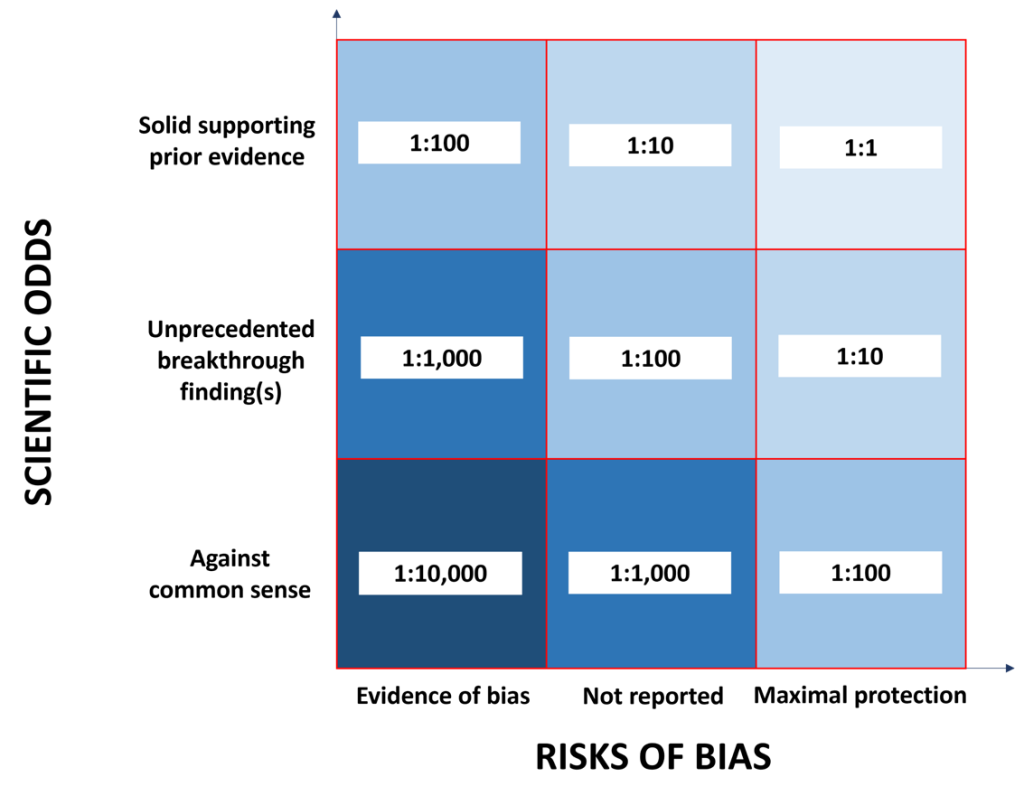
Figure 1: Chances of reported results being true. The indicated odds ratios are a product of scientific odds and technical odds (risks of bias).
Acknowledgment
The author is grateful to the PAASP colleague, Dr. Paul Moser, for stimulating discussions that resulted in the commentary above and the feedback provided on an earlier version.
0 Comments
Leave A Comment